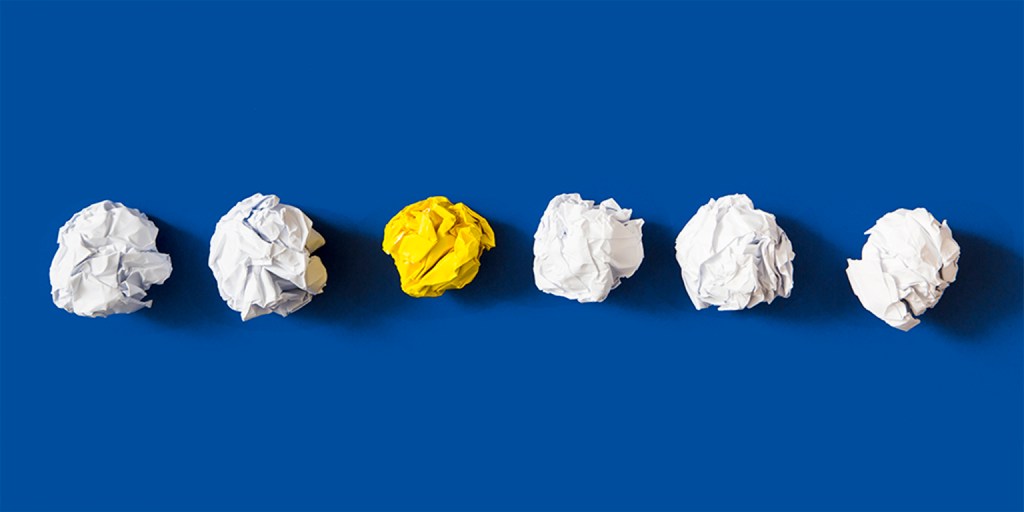
–The Limited Context Understanding of ChatGPT | Image by The Alphabet
Kep Points
- ChatGPT has difficulty retaining extended context during conversations.
- Users may face coherence issues in responses during extensive discussions.
- Employing segmentation and reminders helps mitigate ChatGPT’s context limitation.
ChatGPT’s drawback of limited context understanding pertains to its challenge in retaining and comprehending extended context during interactions. While the model excels in processing individual queries and generating contextually relevant responses, it encounters difficulties in sustaining coherence over prolonged conversations or when handling multiple related queries.
This limitation in maintaining extended context predominantly revolves around its inherent architectural design, notably the fixed-length context windows employed within its processing framework. These context windows represent the model’s method of comprehending and responding to textual input within a predefined range of tokens or words.
In essence, these context windows act as a lens through which ChatGPT perceives and processes information. However, their fixed nature poses a challenge in scenarios where conversations extend beyond the capacity of this limited window. As the discourse progresses, earlier segments of the conversation within this window might fade or become overwritten, impeding the model’s ability to sustain a holistic understanding of the complete conversation thread.
This architectural limitation has implications for ChatGPT’s ability to hold the intricacies and nuances of extended discourse. While it is proficient in handling discrete queries or short exchanges with high coherence, the model’s challenge arises when faced with prolonged or multifaceted interactions. The fixed window restricts the depth and breadth of the context it can retain, leading to potential gaps in continuity or coherence when navigating more extensive conversational landscapes.
How it Arose
The emergence of ChatGPT’s challenge in maintaining extended context primarily stems from the architectural constraints embedded within the model’s design. This limitation arises from the foundational structure of the model, particularly its reliance on fixed-length context windows.
During the training phase, ChatGPT processes textual data in segmented chunks, known as tokens, and comprehends the context within a predefined window. This window acts as a boundary, allowing the model to grasp and retain information within a specified range. However, this mechanism introduces limitations when dealing with extensive or multifaceted conversations.
The model’s architecture necessitates a balance between computational efficiency and contextual understanding. To ensure computational feasibility, the model operates within these fixed context windows, which enable efficient processing but impose limitations on the depth and breadth of retained context. This deliberate choice during the model’s design phase is aimed at optimizing performance without compromising efficiency.
As conversations progress or queries accumulate, earlier portions of the conversation within this fixed context window may exceed its capacity, leading to a displacement or overwrite of previously held context. Consequently, this structural limitation results in the model’s challenge to sustain a comprehensive understanding of the complete conversational flow.
Impact on Users
The limitation in ChatGPT’s ability to maintain extended context significantly affects users engaging in prolonged or multifaceted conversations with the model. This impact manifests in several ways, hindering the quality and coherence of interactions.
In scenarios where users engage ChatGPT in extensive discussions or sequences of related queries, the model’s struggle to retain context becomes evident. As the conversation progresses, earlier portions within the fixed context window might be forgotten or overwritten. Consequently, ChatGPT may produce responses that seem disjointed or fail to adequately consider the full context of the conversation.
For instance, a user engaging ChatGPT in a lengthy discussion about a multifaceted topic, such as geopolitical dynamics, might notice inconsistencies or inaccuracies in the model’s responses. As the discourse expands, the model’s limitation becomes apparent, potentially leading to responses lacking continuity or coherence.
Moreover, users seeking detailed and exhaustive information across a series of interconnected inquiries may experience challenges due to ChatGPT’s difficulty in maintaining comprehensive context. This limitation impacts the overall quality and reliability of the information provided, potentially undermining the trust users place in the model’s responses.
The impact is more pronounced in professional or research settings where a thorough and coherent understanding of an extensive topic is crucial. For example, in academic research, users relying on ChatGPT for in-depth insights may face hurdles in maintaining a coherent and thorough narrative due to the model’s inability to retain the intricate details of an extended discourse.
Mitigation Strategies
Segment Complex Queries: Users can mitigate context loss by breaking down intricate or multipart queries into smaller, more digestible segments. This approach aids ChatGPT in maintaining focus on specific aspects of the conversation, reducing the likelihood of context overload or displacement.
Example: Instead of posing a convoluted question encompassing multiple dimensions, such as “What are the economic, social, and environmental impacts of a policy?” users might segment it into separate, more focused queries targeting each impact individually.
Offer Contextual Reminders: To reinforce ChatGPT’s retention of ongoing discussions, users can interject with contextual reminders referencing key points from previous parts of the conversation. This technique helps in anchoring the model’s understanding and maintaining coherence.
Example: Amidst a discussion about technological challenges, users might periodically interject with statements like, “Regarding the technical obstacles discussed earlier…”
Prompt for Summaries: Encouraging ChatGPT to summarize or synthesize information at strategic intervals serves to underscore critical context. These summaries act as checkpoints, ensuring that the model maintains a cohesive understanding of the ongoing discourse.
Example: Following an elaborate explanation or segment of the conversation, users might prompt ChatGPT with, “Could you summarize the key points discussed so far?”
Explicitly Reiterate Context: Users can guide ChatGPT’s attention explicitly by emphasizing the importance of maintaining ongoing context within the conversation. This approach assists the model in recalling critical elements and fostering coherence.
Example: Before introducing a new segment or query, users might preface it with a reminder, such as “Expanding on our earlier discussion regarding climate change…”
–Introduction to Generative AI | Video by Google Cloud Tech