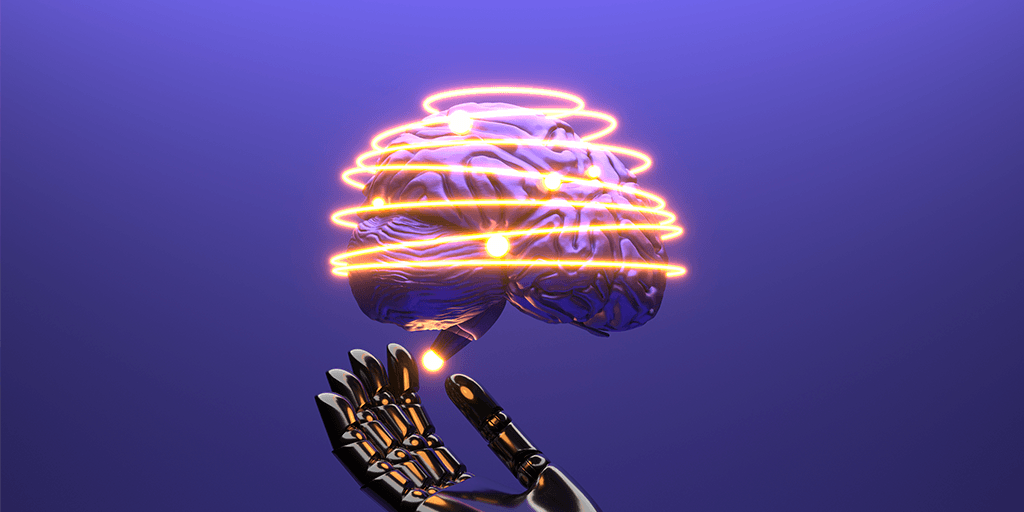
–Swift AI Advancements Challenge Current Benchmarks and Raise Cost, Ethical, and Regulatory Concerns | Image by The Alphabet
Artificial intelligence (AI) systems, exemplified by ChatGPT, are now performing at levels that often meet or surpass human abilities in various domains such as reading comprehension, image recognition, and high-level mathematics, reveals a recent analysis. This swift advancement is rendering traditional benchmarks used for evaluating AI obsolete at an accelerated pace.
This insight comes from the 2024 Artificial Intelligence Index Report, released on April 15 by the Stanford University Institute for Human-Centered Artificial Intelligence in California. The report documents the rapid evolution of machine learning technologies over the last decade.
The report highlights the need for new evaluation methods that can better measure AI capabilities in complex areas like abstract thinking and reasoning. “Benchmarks that once lasted 5–10 years are now outdated within a few years,” states Nestor Maslej, the report’s editor-in-chief and a social scientist at Stanford. He notes the acceleration in improvements has been extraordinarily fast.
Since its initial release in 2017, Stanford’s annual AI Index has been a critical resource for academia and industry alike, providing insights into the technical progress, ethical considerations, and costs associated with AI. The latest edition of the report, which spans over 400 pages and incorporates AI tools in its editing process, also addresses the increasing focus on AI regulation in the U.S. and the challenges in standardizing measures for AI’s responsible use.
Booming
This year’s report also points to a significant increase in AI applications within the scientific community, dedicating a chapter to notable initiatives such as Google DeepMind’s Graph Networks for Materials Exploration and GraphCast for fast weather prediction.
The resurgence in AI research, powered by neural networks and machine learning algorithms, traces back to the early 2010s and has seen exponential growth. For instance, AI projects on GitHub have surged from approximately 800 in 2011 to 1.8 million in the last year, while academic publications on AI have nearly tripled.
The industry is leading the charge in AI innovation, producing 51 significant machine learning systems in the previous year, compared to 15 from academic circles. Academics are increasingly focusing on analyzing and understanding the limitations of these industry-developed models, according to Raymond Mooney, director of the AI Lab at the University of Texas at Austin.
Further challenging these advancements are new, rigorous assessments like the Graduate-Level Google-Proof Q&A Benchmark (GPQA), devised by a group including David Rein from New York University. The GPQA test, featuring over 400 multiple-choice questions, reveals that while PhD scholars answer correctly 65% of questions within their specialty, their success rate drops to 34% on unfamiliar topics, even with internet access. By contrast, AI systems scored between 30–40% last year. This year, Claude 3, a new model from San Francisco-based Anthropic, achieved around 60%. “The pace at which AI is advancing is astonishing,” remarks Rein. “Creating a durable benchmark has become increasingly challenging.”
Concerns
As artificial intelligence (AI) models like OpenAI’s GPT-4 and Google’s Gemini Ultra become more powerful, their associated costs and resource requirements have also surged, raising environmental concerns. Training GPT-4, which was launched in March 2023, reportedly required $78 million, while Gemini Ultra, introduced in December, cost a staggering $191 million. These figures underline the significant energy consumption and water use needed for cooling the data centers that sustain such operations.
According to Nestor Maslej, the development of increasingly large AI models is a primary factor driving these costs. The strategy involves training on vast amounts of text and images, leading to worries about the potential depletion of high-quality training data. For instance, the nonprofit Epoch projected that the world might run out of adequate language data by this year, although a recent update pushes this estimate to 2028.
Beyond the environmental and logistical challenges, there is growing apprehension about the ethical implications of AI development and deployment. Maslej points out that public sentiment towards AI is becoming increasingly wary both in the U.S. and internationally, with attitudes varying significantly by country.
The U.S. has seen a marked increase in regulatory actions concerning AI. From a single AI-referencing regulation in 2016, the count rose to 25 by last year, indicating a sharp uptick in legislative interest post-2022. The focus is now on fostering the responsible use of AI. While new benchmarks are being developed to assess qualities like truthfulness, bias, and likability in AI tools, the lack of standardized metrics complicates efforts to compare and regulate these systems effectively. “Bringing the community together on this is crucial,” Maslej emphasizes, highlighting the importance of unified standards in the evolving landscape of AI governance.
–An index that will track the state of AI over time | Video by Stanford University School of Engineering